Medical Education Transformation
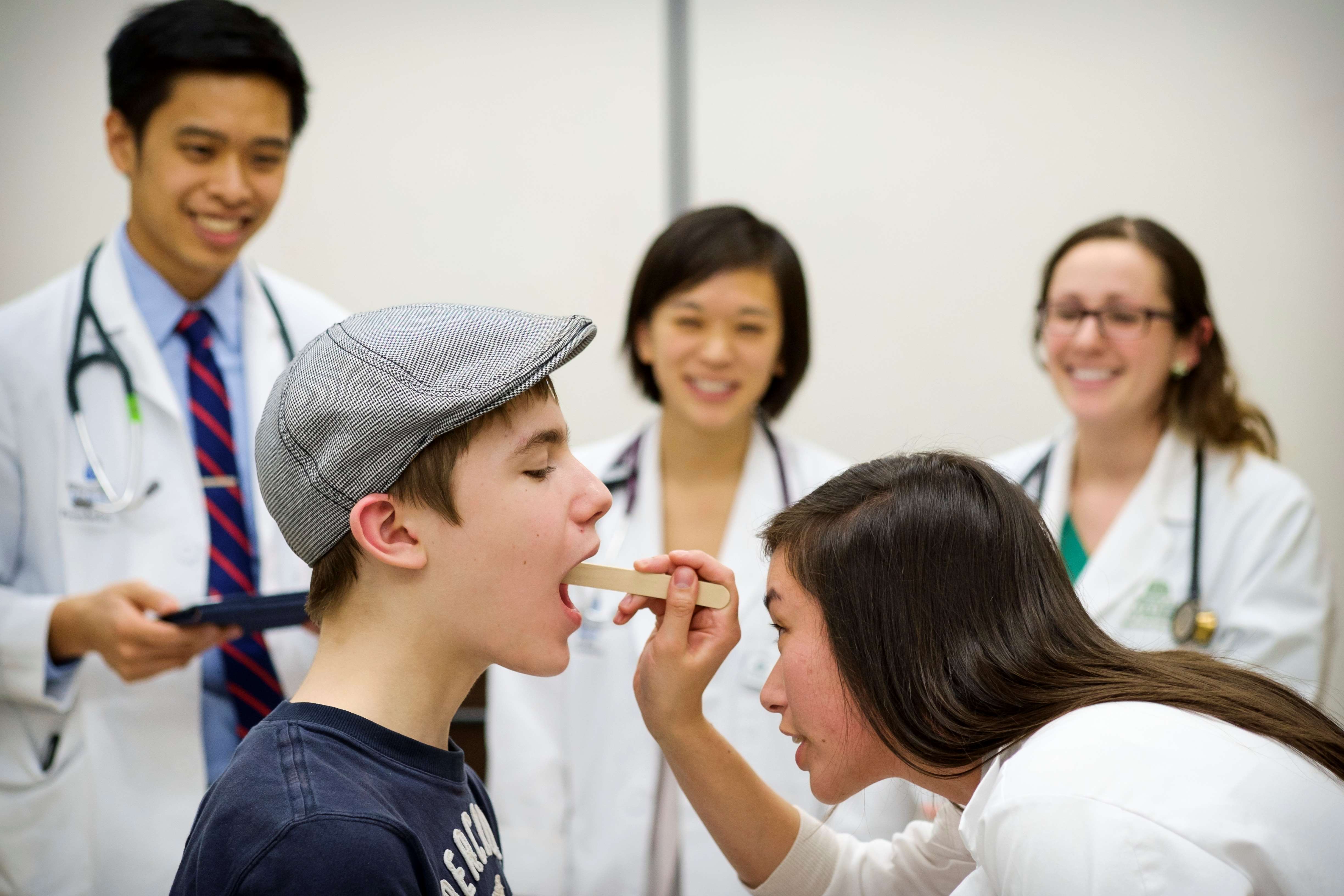
Learn More about our Partnership with the Kern National Network
Learn about how our partnership with the Kern National Network (KNN) and medical schools across the country are creating conditions to ignite a positive culture change that helps individuals, communities and society flourish.Learn More about KNN
Learn More about our Education Mission
Learn about our education mission and the schools, programs and services available, or more information about how we are transforming medical education through our new MCWfusion Curriculum.